Sign up to save your library
With an OverDrive account, you can save your favorite libraries for at-a-glance information about availability. Find out more about OverDrive accounts.
Find this title in Libby, the library reading app by OverDrive.
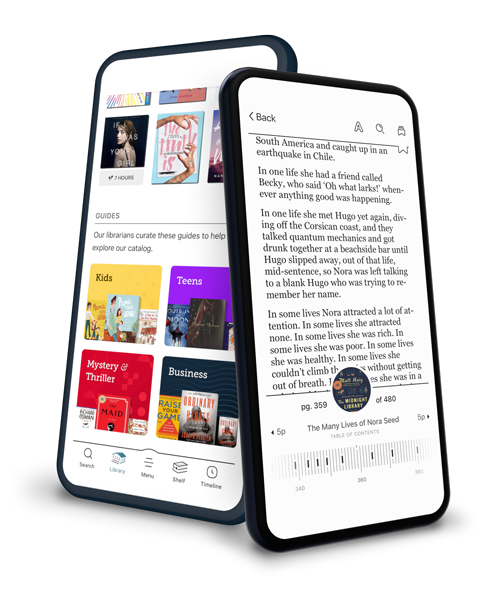
Search for a digital library with this title
Title found at these libraries:
Loading... |
Get the big picture and the important details with this end-to-end guide for designing highly effective, reliable machine learning systems.
From information gathering to release and maintenance, Machine Learning System Design guides you step-by-step through every stage of the machine learning process. Inside, you'll find a reliable framework for building, maintaining, and improving machine learning systems at any scale or complexity.
In Machine Learning System Design: With end-to-end examples you will learn:
The big picture of machine learning system design
Analyzing a problem space to identify the optimal ML solution
Ace ML system design interviews
Selecting appropriate metrics and evaluation criteria
Prioritizing tasks at different stages of ML system design
Solving dataset-related problems with data gathering, error analysis, and feature engineering
Recognizing common pitfalls in ML system development
Designing ML systems to be lean, maintainable, and extensible over time
Authors Valeri Babushkin and Arseny Kravchenko have filled this unique handbook with campfire stories and personal tips from their own extensive careers. You'll learn directly from their experience as you consider every facet of a machine learning system, from requirements gathering and data sourcing to deployment and management of the finished system.
About the technology
Designing and delivering a machine learning system is an intricate multistep process that requires many skills and roles. Whether you're an engineer adding machine learning to an existing application or designing a ML system from the ground up, you need to navigate massive datasets and streams, lock down testing and deployment requirements, and master the unique complexities of putting ML models into production. That's where this book comes in.
About the book
Machine Learning System Design shows you how to design and deploy a machine learning project from start to finish. You'll follow a step-by-step framework for designing, implementing, releasing, and maintaining ML systems. As you go, requirement checklists and real-world examples help you prepare to deliver and optimize your own ML systems. You'll especially love the campfire stories and personal tips, and ML system design interview tips.
What's inside
Metrics and evaluation criteria
Solve common dataset problems
Common pitfalls in ML system development
ML system design interview tips
About the reader
For readers who know the basics of software engineering and machine learning. Examples in Python.
About the author
Valerii Babushkin is an accomplished data science leader with extensive experience. He currently serves as a Senior Principal at BP. Arseny Kravchenko is a seasoned ML engineer currently working as a Senior Staff Machine Learning Engineer at Instrumental.
Table of Contents
Part 1
1 Essentials of machine learning system design
2 Is there a problem?
3 Preliminary research
4 Design document
Part 2
5 Loss functions and metrics
6 Gathering datasets
7 Validation schemas
8 Baseline solution
Part 3
9 Error analysis
10 Training pipelines
11 Features and feature engineering
12 Measuring and reporting results
Part 4
13 Integration
14 Monitoring and reliability
15 Serving and inference optimization
16 Ownership and maintenance
From information gathering to release and maintenance, Machine Learning System Design guides you step-by-step through every stage of the machine learning process. Inside, you'll find a reliable framework for building, maintaining, and improving machine learning systems at any scale or complexity.
In Machine Learning System Design: With end-to-end examples you will learn:
Authors Valeri Babushkin and Arseny Kravchenko have filled this unique handbook with campfire stories and personal tips from their own extensive careers. You'll learn directly from their experience as you consider every facet of a machine learning system, from requirements gathering and data sourcing to deployment and management of the finished system.
About the technology
Designing and delivering a machine learning system is an intricate multistep process that requires many skills and roles. Whether you're an engineer adding machine learning to an existing application or designing a ML system from the ground up, you need to navigate massive datasets and streams, lock down testing and deployment requirements, and master the unique complexities of putting ML models into production. That's where this book comes in.
About the book
Machine Learning System Design shows you how to design and deploy a machine learning project from start to finish. You'll follow a step-by-step framework for designing, implementing, releasing, and maintaining ML systems. As you go, requirement checklists and real-world examples help you prepare to deliver and optimize your own ML systems. You'll especially love the campfire stories and personal tips, and ML system design interview tips.
What's inside
About the reader
For readers who know the basics of software engineering and machine learning. Examples in Python.
About the author
Valerii Babushkin is an accomplished data science leader with extensive experience. He currently serves as a Senior Principal at BP. Arseny Kravchenko is a seasoned ML engineer currently working as a Senior Staff Machine Learning Engineer at Instrumental.
Table of Contents
Part 1
1 Essentials of machine learning system design
2 Is there a problem?
3 Preliminary research
4 Design document
Part 2
5 Loss functions and metrics
6 Gathering datasets
7 Validation schemas
8 Baseline solution
Part 3
9 Error analysis
10 Training pipelines
11 Features and feature engineering
12 Measuring and reporting results
Part 4
13 Integration
14 Monitoring and reliability
15 Serving and inference optimization
16 Ownership and maintenance